Pythonを用いた多層ニューラルネットワークのプログラムの実装
Pythonを使った全結合層のみで構成される多層ニューラルネットワークのプログラムの生成
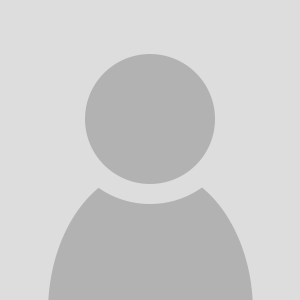
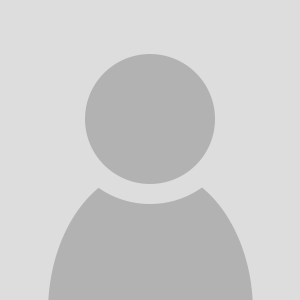
投稿日時:
- プロンプト実行例
- プロンプトを見る
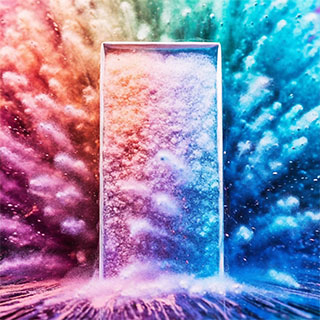
Hello 教えて.AI !!
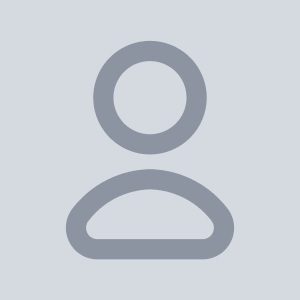
あなたはPythonを活用した精通したAIプログラマです。 全結合層のみで構成された多層ニューラルネットワークのプログラムを作成してください。
#制約条件: ・正解率、適合率、再現率、F値を算出するプログラムにすること ・グラフで可視化できるプログラムも追記すること ・データセットは、scikit-learnのサンプルデータセットである「load_breast_cancer」を利用すること
#プログラムで用いるハイパーパラメータ: ・重みの初期値の標準偏差を指定 weight_init_std = 0.05
・ 試行するエポック数を指定 max_epochs = 20
・全データ数に対するバッチデータ数の割合(エポック数判定用) iter_per_epoch = max(train_size / batch_size, 1)
・試行エポック数を初期化 epoch_cnt = 0
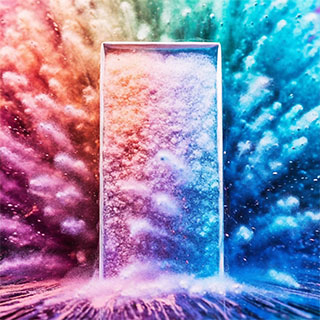
import numpy as np from sklearn.datasets import load_breast_cancer from sklearn.model_selection import train_test_split import matplotlib.pyplot as plt
データセットの読み込み
breast_cancer = load_breast_cancer() X, y = breast_cancer.data, breast_cancer.target
データセットの分割
X_train, X_test, y_train, y_test = train_test_split(X, y, test_size=0.2, random_state=42)
ハイパーパラメータの設定
weight_init_std = 0.05 max_epochs = 20 batch_size = 32 iter_per_epoch = max(len(X_train) / batch_size, 1) epoch_cnt = 0
シグモイド関数
def sigmoid(x): return 1 / (1 + np.exp(-x))
ReLU関数
def relu(x): return np.maximum(0, x)
ソフトマックス関数
def softmax(x): exp_x = np.exp(x) return exp_x / np.sum(exp_x, axis=1, keepdims=True)
交差エントロピー誤差
def cross_entropy_error(y_pred, y_true): delta = 1e-7 return -np.mean(np.sum(y_true * np.log(y_pred + delta), axis=1))
正解率、適合率、再現率、F値の算出
def calculate_metrics(y_pred, y_true): y_pred_labels = np.argmax(y_pred, axis=1) y_true_labels = np.argmax(y_true, axis=1) accuracy = np.mean(y_pred_labels == y_true_labels)
1true_positive = np.sum((y_pred_labels == 1) & (y_true_labels == 1)) 2false_positive = np.sum((y_pred_labels == 1) & (y_true_labels == 0)) 3false_negative = np.sum((y_pred_labels == 0) & (y_true_labels == 1)) 4 5precision = true_positive / (true_positive + false_positive) 6recall = true_positive / (true_positive + false_negative) 7f1_score = 2 * (precision * recall) / (precision + recall) 8 9return accuracy, precision, recall, f1_score
ニューラルネットワーククラス
class MLP: def init(self, input_size, hidden_size, output_size): self.params = {} self.params['W1'] = weight_init_std * np.random.randn(input_size, hidden_size) self.params['b1'] = np.zeros(hidden_size) self.params['W2'] = weight_init_std * np.random.randn(hidden_size, output_size) self.params['b2'] = np.zeros(output_size)
1def forward(self, x): 2 z1 = np.dot(x, self.params['W1']) + self.params['b1'] 3 a1 = relu(z1) 4 z2 = np.dot(a1, self.params['W2']) + self.params['b2'] 5 y_pred = softmax(z2) 6 return y_pred 7 8def backward(self, x, y_true, y_pred): 9 batch_size = x.shape[0] 10 11 # 出力層の誤差 12 delta2 = (y_pred - y_true) / batch_size 13 14 # 重みとバイアスの勾配 15 grads = {} 16 grads['W2'] = np.dot(self.hidden_layer.T, delta2) 17 grads['b2'] = np.sum(delta2, axis=0) 18 19 # 隠れ層の誤差 20 delta1 = np.dot(delta2, self.params['W2'].T) * (self.hidden_layer > 0) 21 22 # 重みとバイアスの勾配 23 grads['W1'] = np.dot(x.T, delta1) 24 grads['b1'] = np.sum(delta1, axis=0) 25 26 return grads 27 28def train(self, x, y_true): 29 # 順伝播 30 y_pred = self.forward(x) 31 32 # バックプロパゲーション 33 grads = self.backward(x, y_true, y_pred) 34 35 # パラメータの更新 36 for key in self.params.keys(): 37 self.params[key] -= learning_rate * grads[key] 38 39def predict(self, x): 40 y_pred = self.forward(x) 41 return np.argmax(y_pred, axis=1)
多層ニューラルネットワークモデルの作成
model = MLP(input_size=X_train.shape[1], hidden_size=50, output_size=2)
学習
train_loss_list = [] train_acc_list = [] test_acc_list = []
for epoch in range(max_epochs): # エポックごとにシャッフル idx = np.random.permutation(len(X_train)) X_train = X_train[idx] y_train = y_train[idx]
1for i in range(0, len(X_train), batch_size): 2 # バッチデータの取得 3 X_batch = X_train[i:i+batch_size] 4 y_batch = y_train[i:i+batch_size] 5 6 # バッチデータで学習 7 model.train(X_batch, y_batch) 8 9# エポックごとの評価 10train_pred = model.forward(X_train) 11test_pred = model.forward(X_test) 12train_loss = cross_entropy_error(train_pred, y_train) 13train_acc, _, _, _ = calculate_metrics(train_pred, y_train) 14test_acc, _, _, _ = calculate_metrics(test_pred, y_test) 15 16train_loss_list.append(train_loss) 17train_acc_list.append(train_acc) 18test_acc_list.append(test_acc) 19 20epoch_cnt += 1 21if epoch_cnt >= iter_per_epoch: 22 print("Epoch:", epoch+1) 23 print("Train Loss:", train_loss) 24 print("Train Accuracy:", train_acc) 25 print("Test Accuracy:", test_acc) 26 epoch_cnt = 0
グラフの描画
plt.plot(range(1, max_epochs+1), train_loss_list, label='Train Loss') plt.xlabel('Epoch') plt.ylabel('Loss') plt.legend() plt.show()
plt.plot(range(1, max_epochs+1), train_acc_list, label='Train Accuracy') plt.plot(range(1, max_epochs+1), test_acc_list, label='Test Accuracy') plt.xlabel('Epoch') plt.ylabel('Accuracy') plt.legend() plt.show()
コメント 0
他のプロンプトもチェック
音楽
推奨モデル - GPT-4oテーマを入力するだけで、韻を踏んだラップの歌詞を簡単・直感的に作成できます。あなたのオリジナルヒット曲が、このプロンプトから生まれるかもしれません。 特徴とメリット: 即効性: 簡単な操作で、テーマに合った韻を踏んだ歌詞が即座に生成されます。 SunoAI最適化: 「Suno AI」を利用した音楽制作に最適化されており、あなたの曲にぴったりなリリックを作り上げます。 クリエイティブな自由度: 提供された歌詞は自由に編集・アレンジが可能。あなたの独自のスタイルを反映させることができます。 😊少しでも気に入っていただけましたら、お気に入り(❤マーク)をクリックしていただけると、とても励みになりますので、ぜひお願いいたします。また、「いつか使ってみよう」と思っている方も、お気に入りに登録しておくと、いつでもマイページからすぐにご利用いただけます。 🌟 SunoAIについて SunoAIを使うと誰でも簡単に作曲ができます。 https://app.suno.ai/ 使い方はYouTubeなどで検索してみてください。30921.21KGPTs
キャラクター作成
「走るパンダ」「月の下で踊るパンダ」のように、お好きなテーマを入力するだけ! あとはAIが素敵な「アールヌーボー様式」のイラストに変換してくれます🐼 オプションで「アールデコ様式」も選べます801280物語・脚本・プロット
推奨モデル - GPT-4o入力されたキーワードをもとに進次郎構文を生成します。 クスッとしたい方必見です🤙 #小泉進次郎 #進次郎構文10582.81K